GridGain helps customers scale their business applications across numerous industries transforming to digital business models, including financial services, logistics, software, healthcare, retail, telecommunications and more.
Sample Customer List
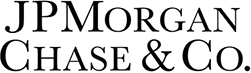
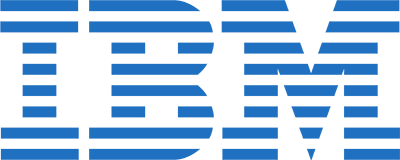
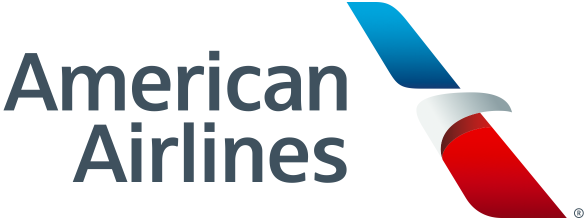
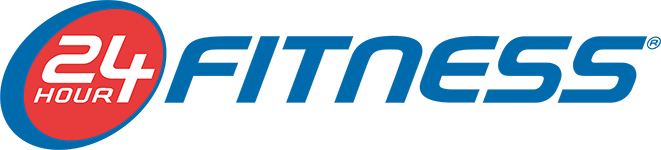
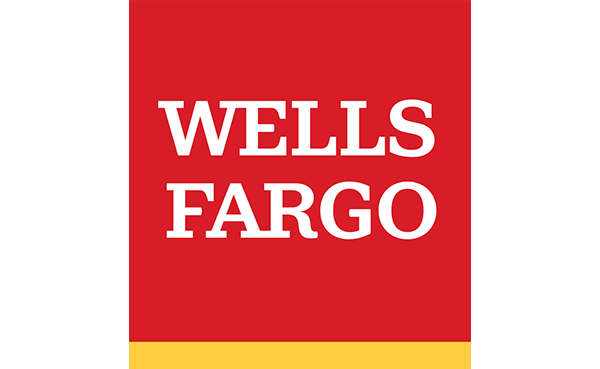
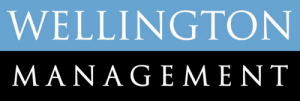
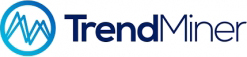
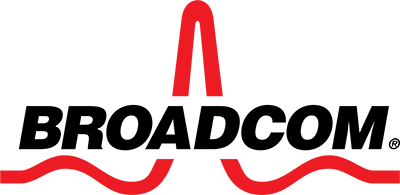
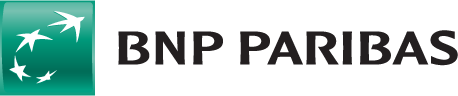

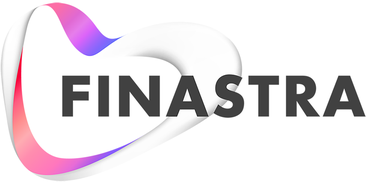
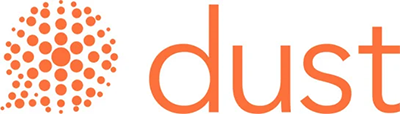
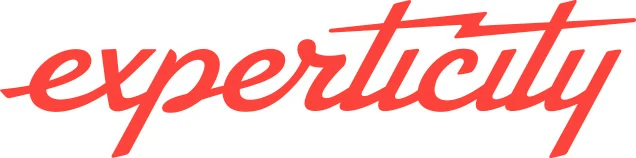


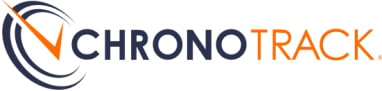
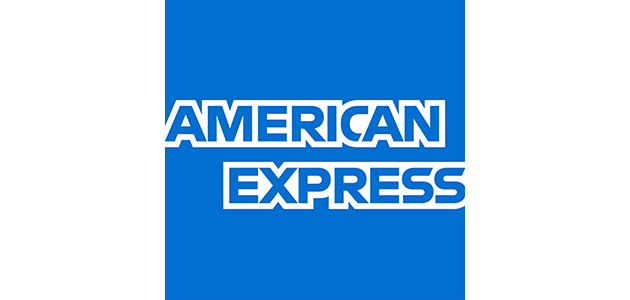

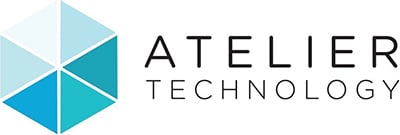
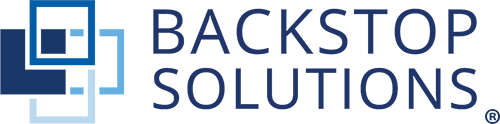
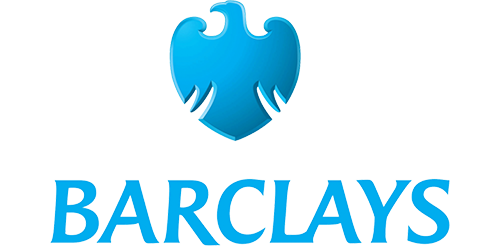
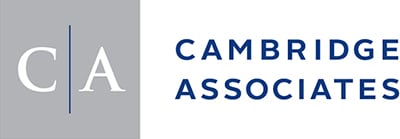
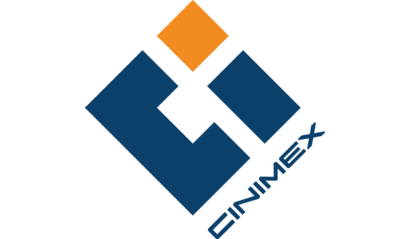
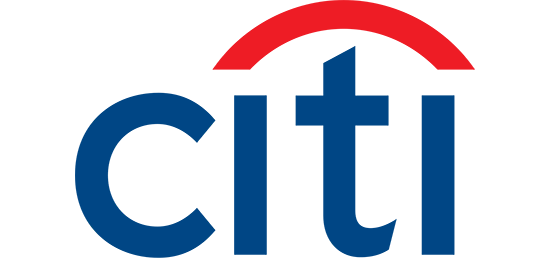
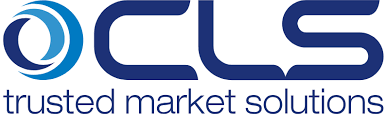
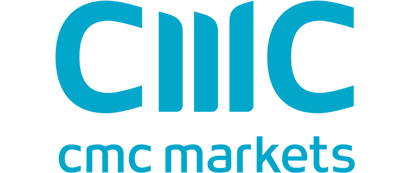
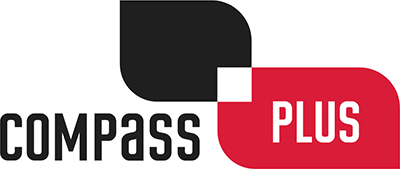
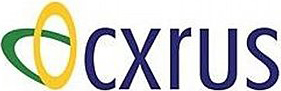

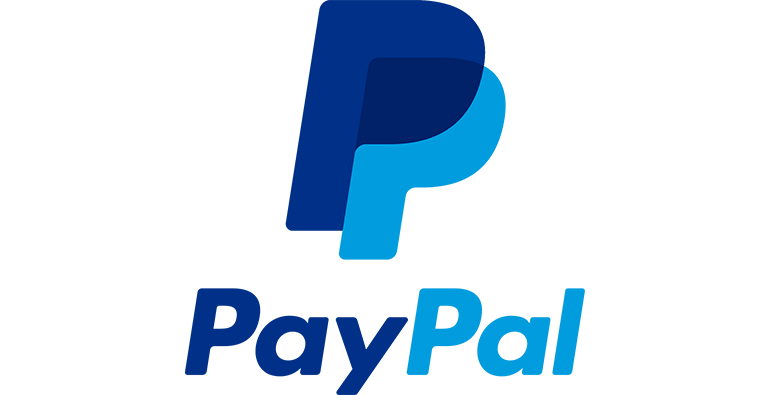
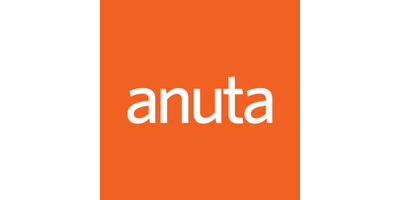

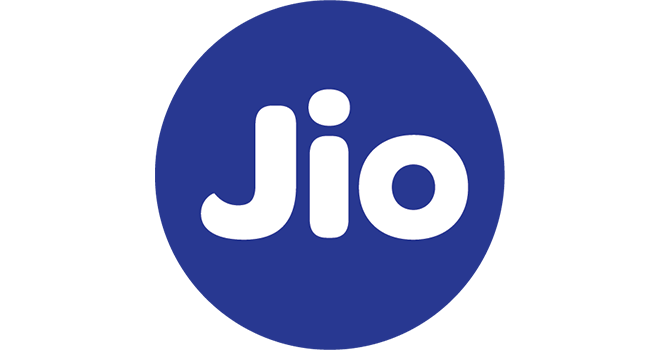
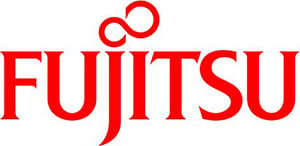
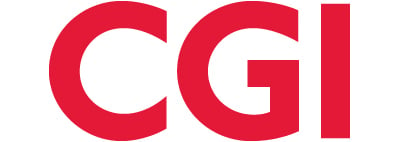
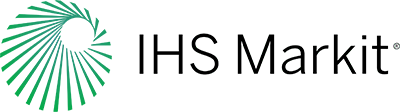
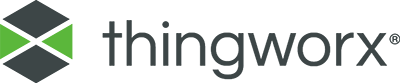
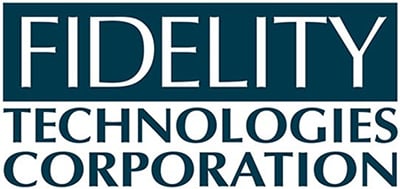
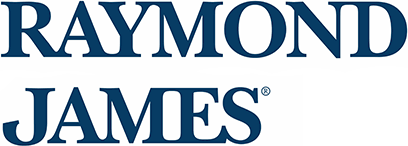
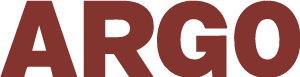
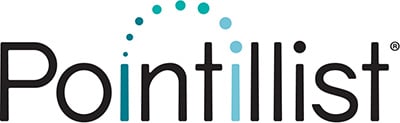
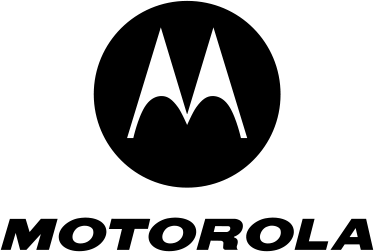
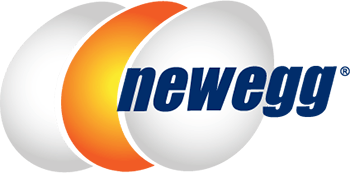
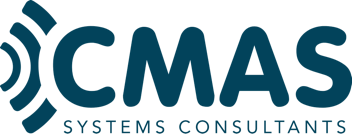
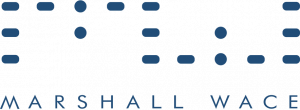

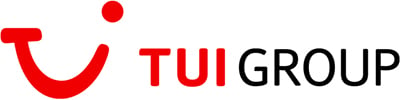
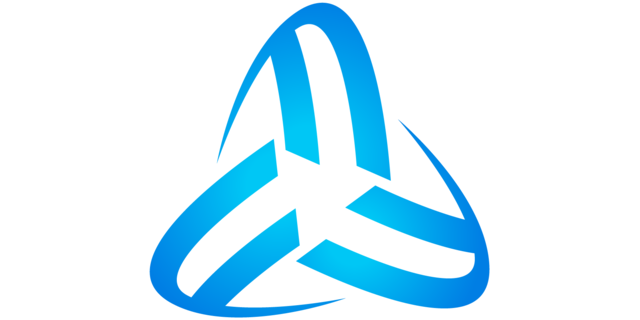
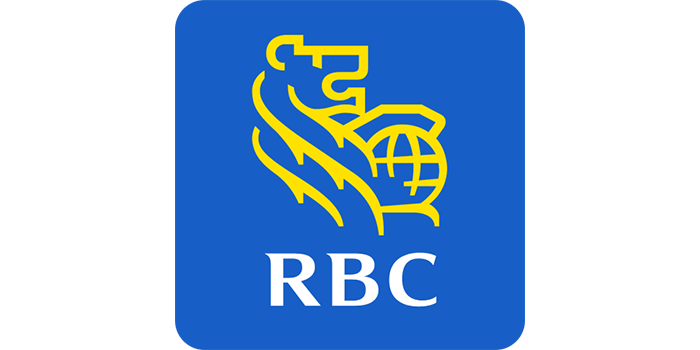
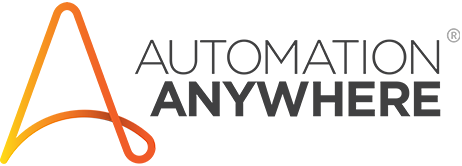
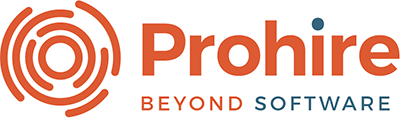
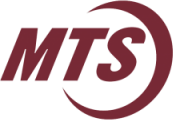
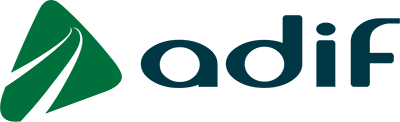
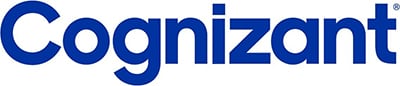
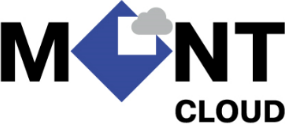
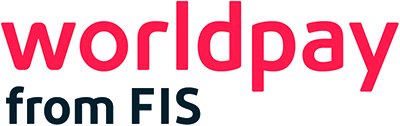
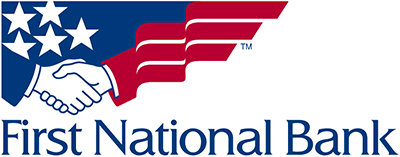
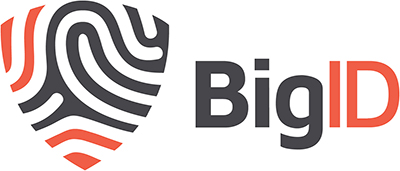
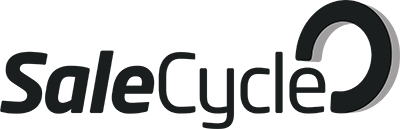
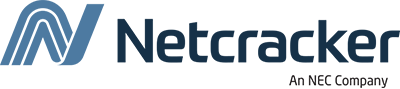
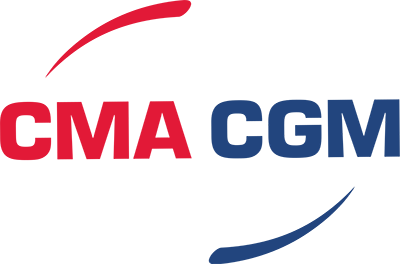
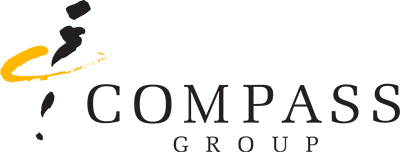
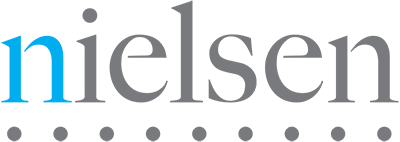
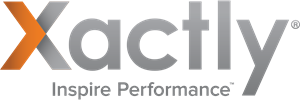
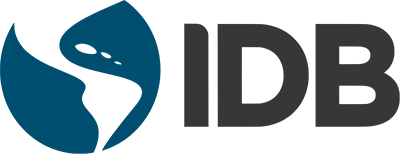
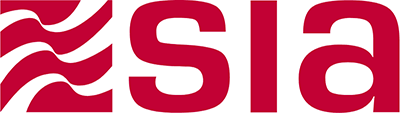


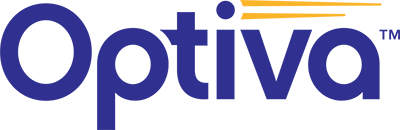
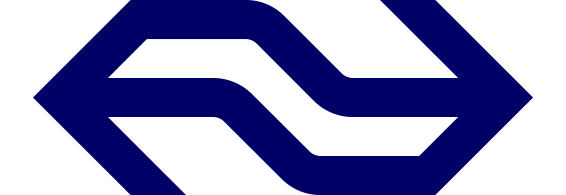
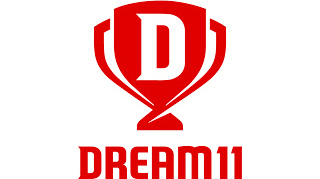
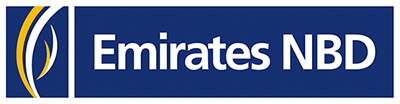
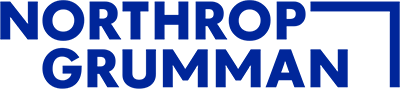

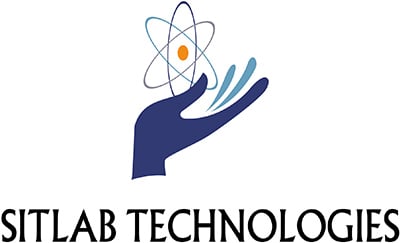
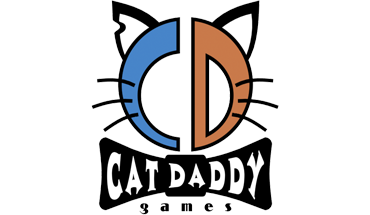


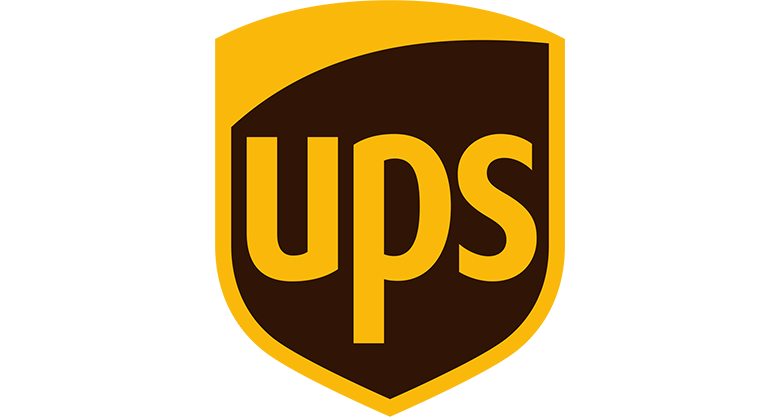
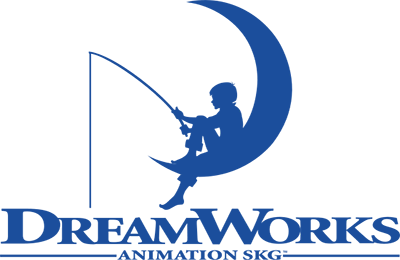
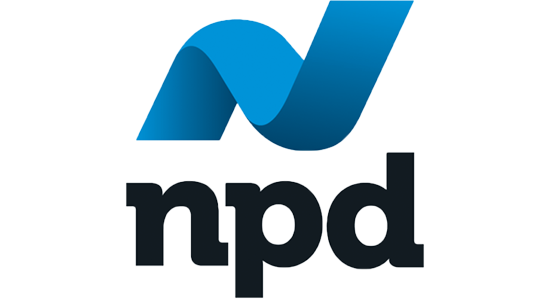
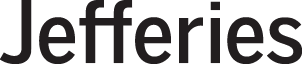

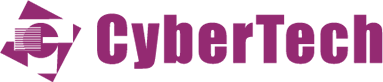
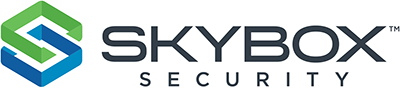

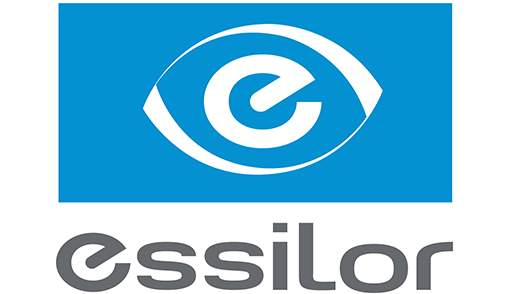
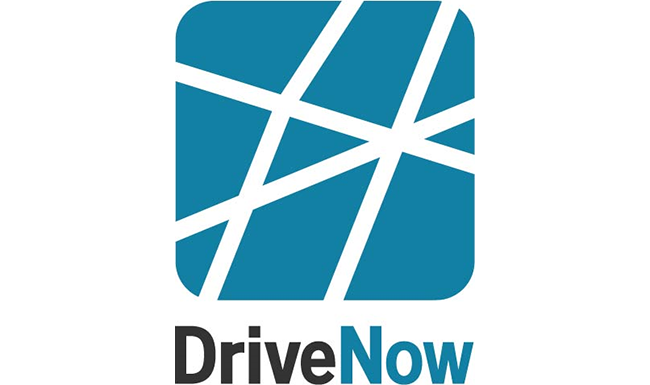
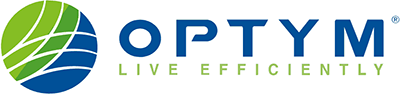
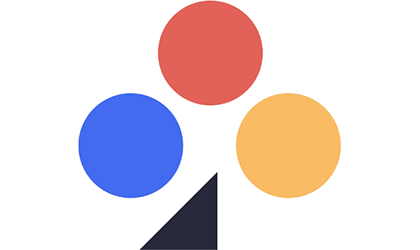

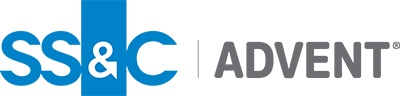
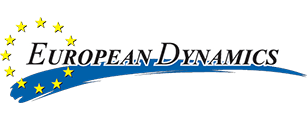
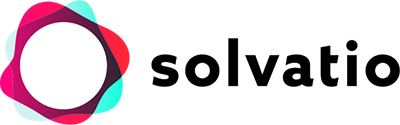

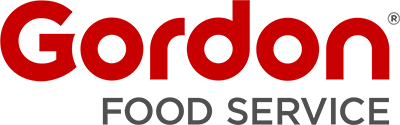
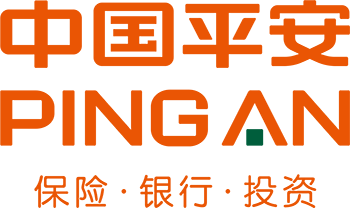
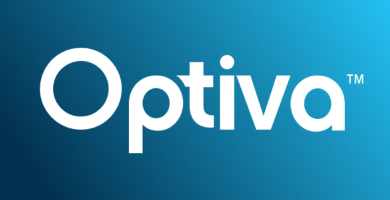

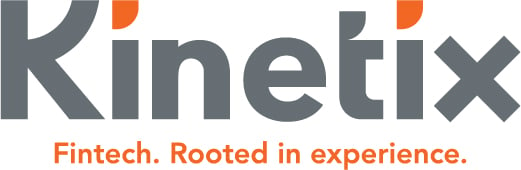
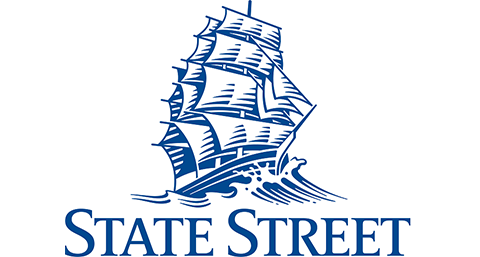
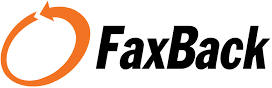

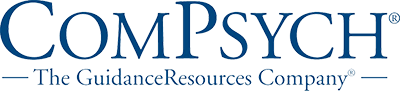

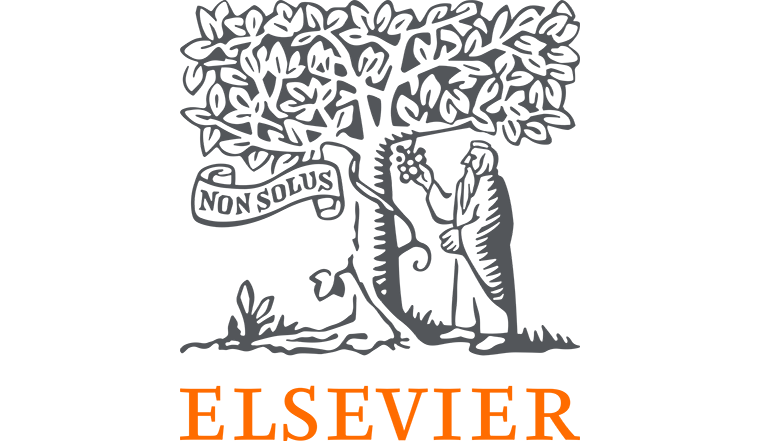
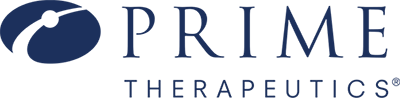
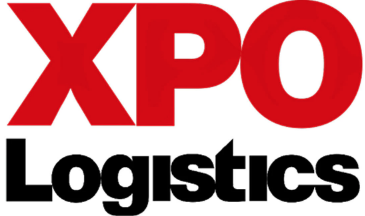
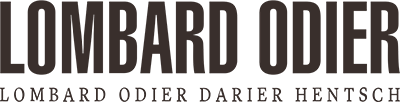
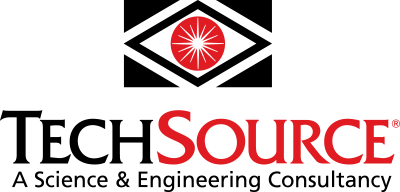
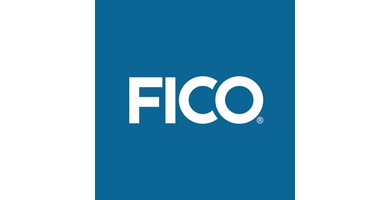
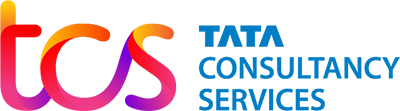

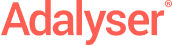
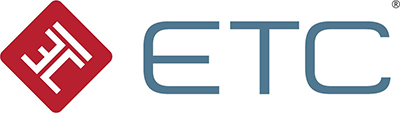

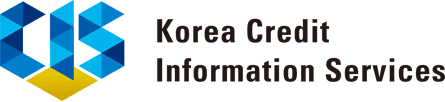
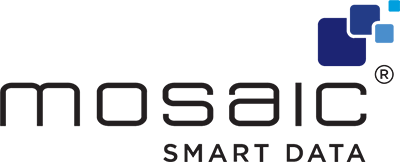
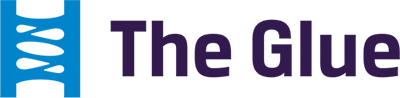
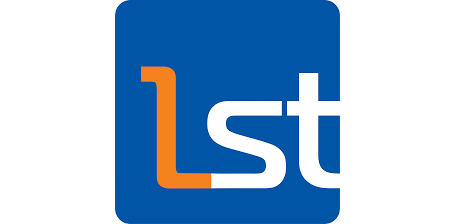

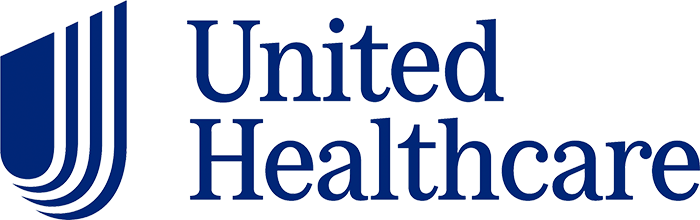
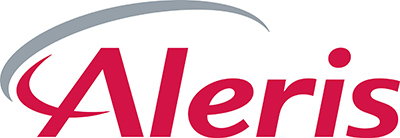

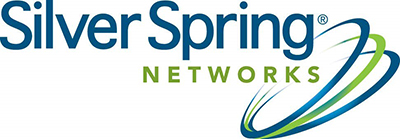
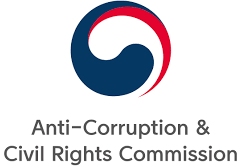

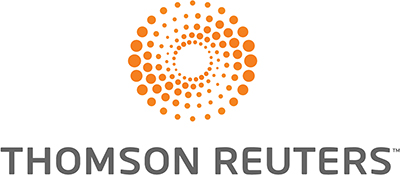


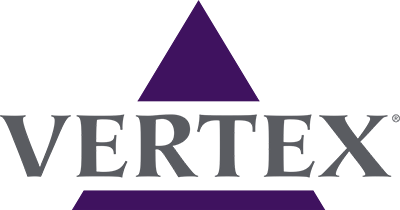
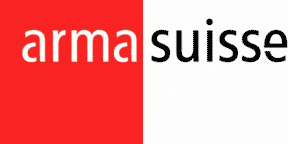
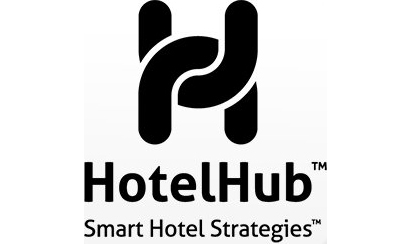
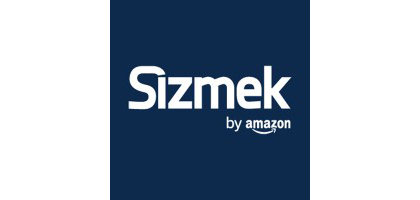

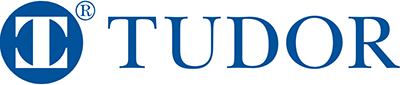

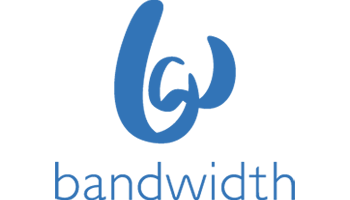
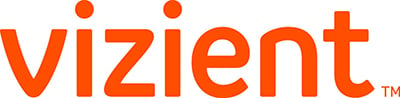

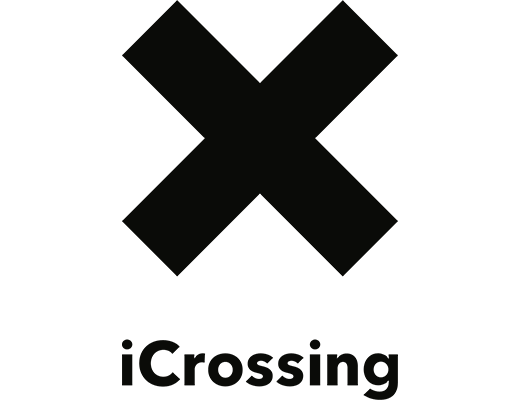
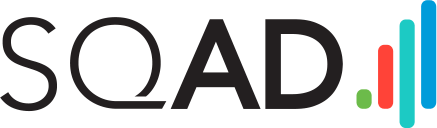
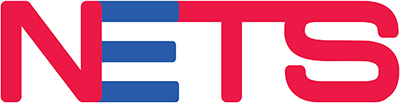
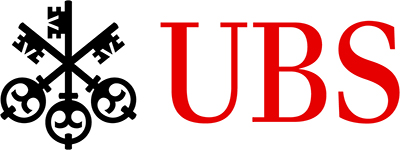
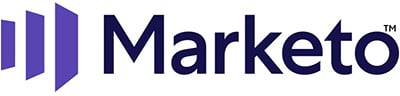
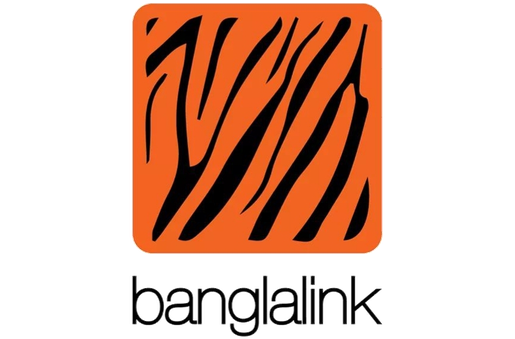

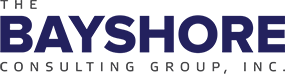

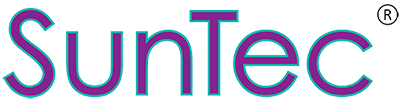
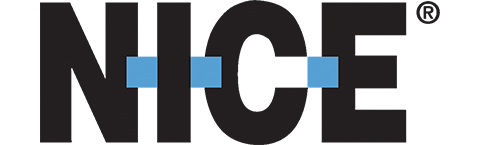


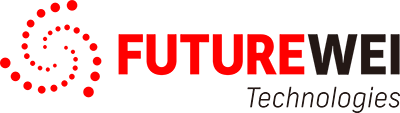
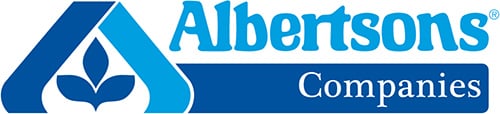
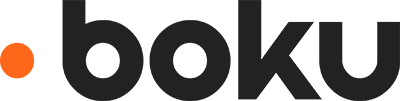
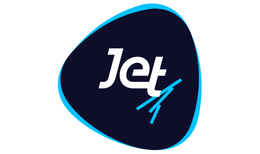

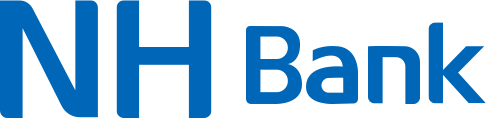
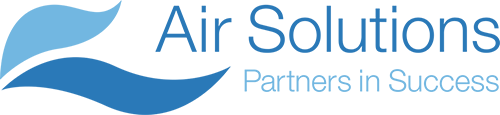
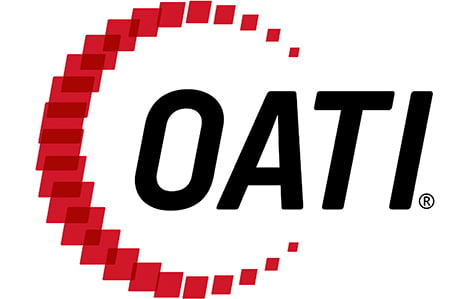
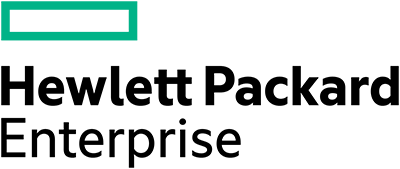
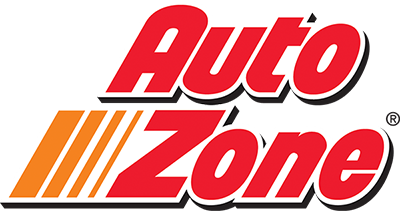


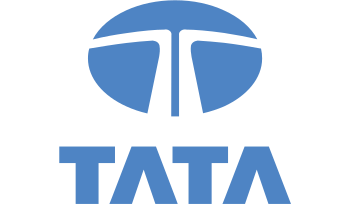
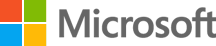
GridGain helps customers scale their business applications across numerous industries transforming to digital business models, including financial services, logistics, software, healthcare, retail, telecommunications and more.