In-Memory Cache
What is an In-Memory Cache?
An in-memory cache is a data storage layer that sits between applications and databases to deliver responses with high speeds by storing data from earlier requests or copied directly from databases. An in-memory cache removes the performance delays when an application built on a disk-based database must retrieve data from a disk before processing.
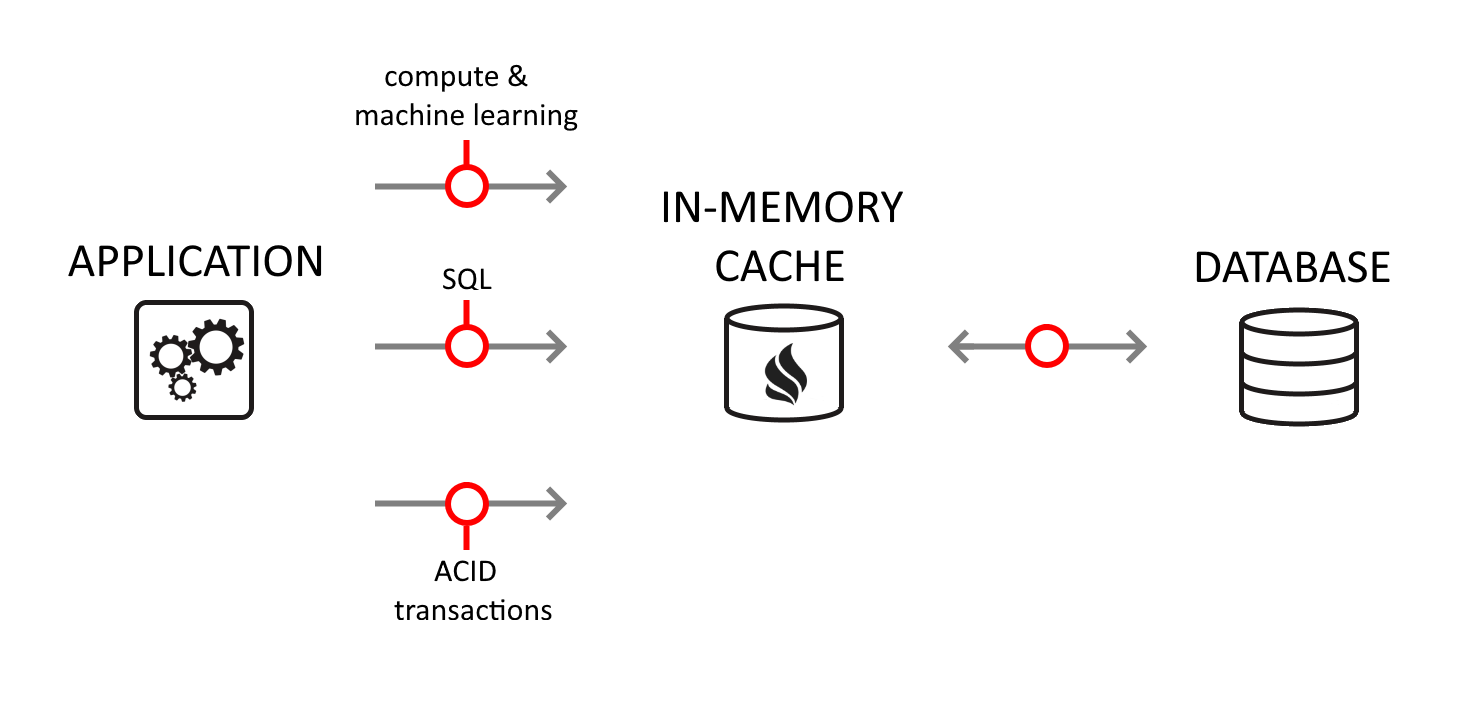
Reading data from memory is faster than from the disk. In-memory caching avoids latency and improves online application performance. Background jobs that took hours or minutes can now execute in minutes or seconds.
Since cache data is kept separate from the application, the in-memory cache requires data movement from the cache system to the application and back to the cache for processing. This often occurs across networks.
Distributed Versus Non-Distributed
Developers typically deploy caching solutions, such as Memcache, on a single node. Still, these solutions can’t scale to meet today’s big data demands. This is due to the physical limitations in a single server’s RAM.
Distributed caching enables multiple nodes to work together to hold massive amounts of cached data. This caching method breaks a large data set into shards and distributes them across all nodes of the in-memory computing cluster.
Caching Strategies
Depending on how often you update your database and whether consistency is a bigger concern than speed, you can choose from various cache strategies: cache aside, read-through, and write-through.
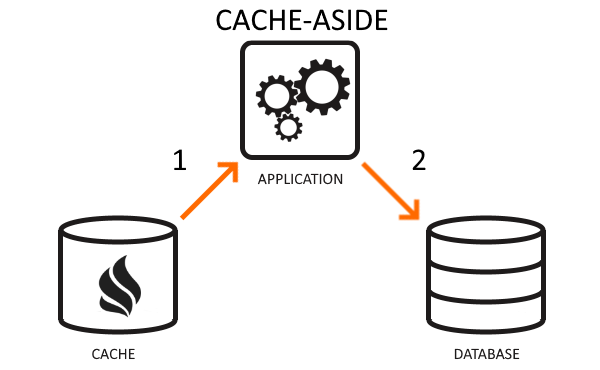
Cache-aside is the standard, simple caching strategy. The application lazy-loads database data on-demand to boost data fetching performance. In this strategy, the application first requests the data from the cache. If data exists in the cache (when a “cache hit” happens), the app retrieves information from the cache. If data is not in the cache (a “cache miss”), the app reads data from the data store and inserts it into or updates it to the cache for later use.
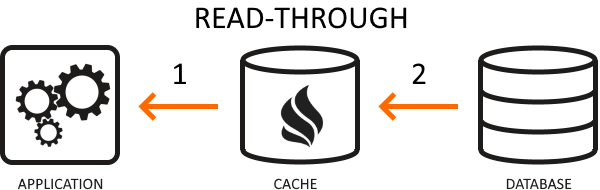
Read-through is a form of lazy-load caching similar to the cache-aside strategy. However, unlike cache-aside, your app is unaware of the data store. So, it queries the database by the caching mechanism instead. By delegating database reads to the cache component, your application code becomes cleaner — but at the expense of configuring extra caching components for database credentials.
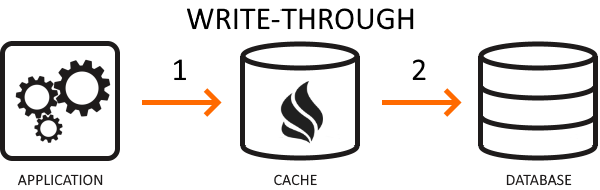
Write-through is a caching strategy where your application delegates database operations to the cache (similarly to the read-through cache, but for writes). Your app updates the cache, which then synchronously writes the new data to the database. This process slows the writing operation but ensures cache consistency and reliability. Developers use this strategy when consistency is a significant concern, and the application doesn’t frequently write data to the cache.
Real-World Use
According to the results of a study released by Google in 2016, 53 percent of visitors abandon a website if it takes longer than three seconds to load. As a result, enterprises increasingly look for solutions such as in-memory cache to improve application performance.
Accelerating online database applications is the most common use case for in-memory caching. For example, a high-traffic website storing content in a database will significantly benefit from the in-memory cache. The website can replace frequent, time-consuming, disk-based database reads and writes with faster and reliable operations based on memory for online transactions.
Another related use case is a business intelligence (BI) report requiring complex queries to a data store. When business users check the report frequently, but it contains seldomly-updated historical data, a cache sitting in front of the data store can save time, network bandwidth, and processing capacity.
Apache Ignite and GridGain
Apache Ignite is a distributed database for high-performance computing with in-memory speed. Application developers frequently select it for the caching use case. As a distributed in-memory cache, Ignite supports a wide array of developer APIs including SQL queries, key-value, compute, and ACID transactions.
The open-source Apache Ignite code forms the foundation of GridGain. The enterprise-grade GridGain In-Memory Computing Platform allows deployment of Ignite securely at global scale with zero downtime.
Conclusion
In recent years, businesses have been moving their in-store experiences to real-time digital interactions, spiking interest in in-memory cache computing. But, we developers understand that technology alone isn’t enough for a business to succeed: We need to stay informed about which architecture, tools, and services adequately address our company’s in-memory distributed caching needs. We need to be aware of new approaches that deliver real-time experiences without disrupting internal systems or requiring developers to switch to a completely new technical stack.
As the leading edge of in-memory computing, Apache Ignite and GridGain help us address those needs. Learn more about how to leverage the power of Apache Ignite, access the GridGain In-Memory Computing Platform, solve your distributed caching challenges, and become production-ready and cloud-native with GridGain.
Ready to learn more?
Explore our products and services →