Turbocharge Your SQL Queries In-Memory with Apache Ignite™
About
Join Nikita Ivanov, CTO of GridGain Systems and member of the Project Committee for Apache Ignite, to learn how to boost performance 1,000x and scale to over 1 billion transactions per second with in-memory storage of hundreds of TBs of data for your SQL-based applications.
Nikita will show how Apache Ignite handles auto-loading of SQL schema and data, SQL indexes, compound indexes support, and various forms of SQL queries including distributed SQL joins, joins across caches, predicate-based queries, queries over sliding windows for streaming data, and more.
He will also take live questions from the audience, so this is your opportunity to learn how to accelerate your SQL-based applications directly from a foremost expert in the field.
Speakers
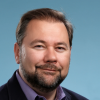
Nikita Ivanov
Founder & CTO, GridGain Systems
Founder & CTO, GridGain Systems