The Emergence of Converged Data Platforms and the Role of In-Memory Computing
Organizations today typically have heterogeneous IT infrastructures which include a variety of database technologies and a wide variety of applications drawing on that data. Many organizations are dealing with massive and rapidly growing amounts of data with end users requiring immediate access to this real-time big data for both transactional and BI applications. Technology providers are responding to these evolving needs by moving towards a converged data platform which includes:
- A distributed grid/cache
- NoSQL database integration
- Relational operational database integration
- Analytic database integration
- Hadoop integration
- Stream processing
- Containerization
In this webinar, Matt Aslett from 451 Research will discuss the drivers behind the need for a converged data platform and the current state of the evolution of these solutions.
Nikita Ivanov from GridGain Systems will then discuss the role of in-memory computing within the converged data platform and describe example use cases.
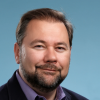
Founder & CTO, GridGain Systems
Nikita Ivanov is founder and CTO of GridGain Systems, started in 2007 and funded by RTP Ventures and Almaz Capital. Nikita provides the vision and leadership at GridGain to develop the world’s top in-memory computing platform, now used by thousands of organizations around the globe to power business-critical systems and enable digital transformation initiatives.
Nikita has over 20 years of experience in software application development, building HPC and middleware platforms, and contributing to the efforts of other startups and notable companies including Adaptec, Visa and BEA Systems. Nikita was one of the pioneers in using Java technology for server side middleware development while working for one of Europe’s largest system integrators in 1996.
He is an active member of Java middleware community, contributor to the Java specification, and holds a Master’s degree in Electro Mechanics from the Baltic State Technical University, Saint Petersburg, Russia.