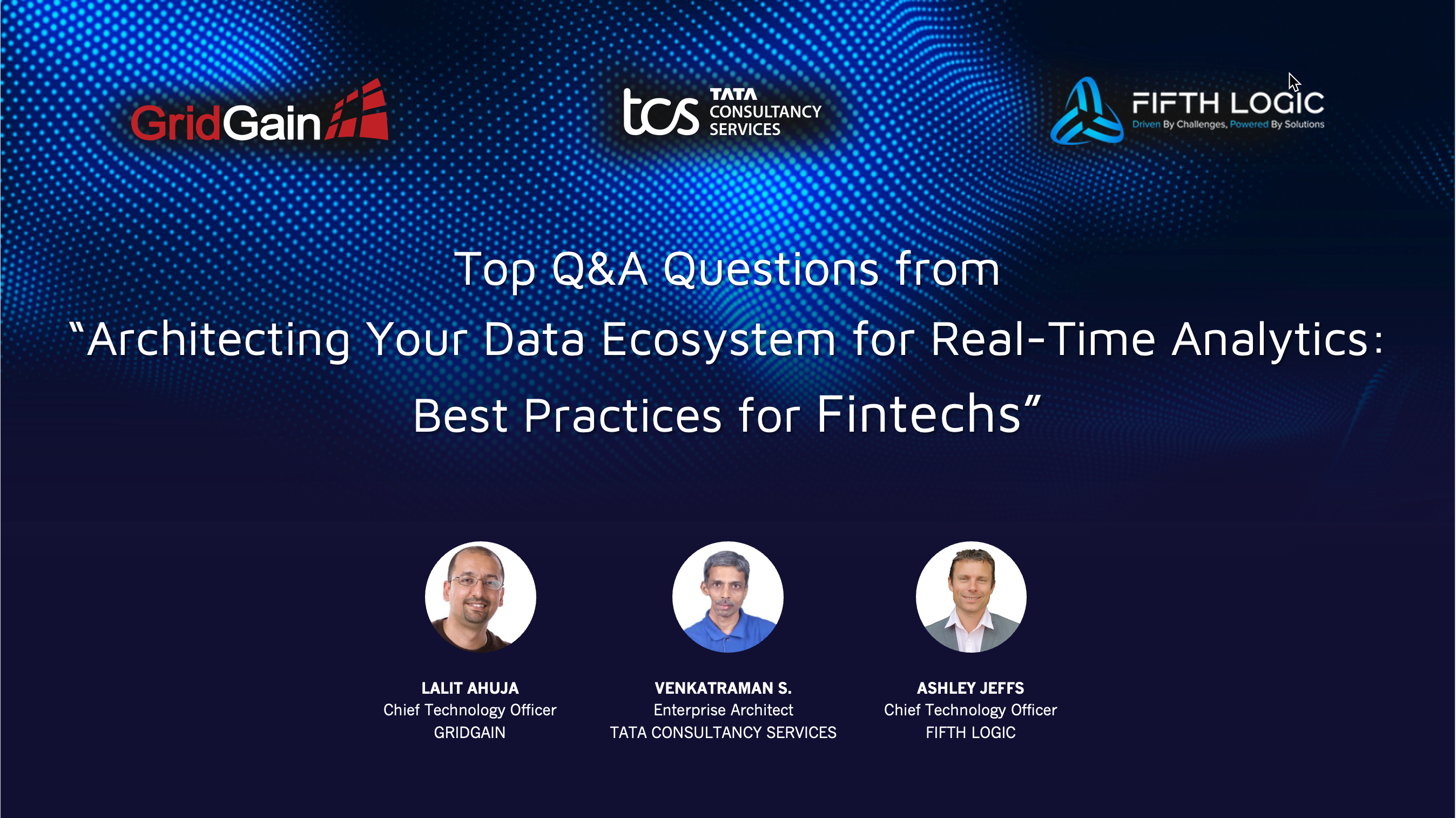
We hosted a rich technical conversation around the data challenges faced by Fintechs on our recent webinar, “Architecting Your Data Ecosystem for Real-Time Analytics: Best Practices for Fintechs.” This included many great questions from our audience during the Q&A.
Here is a recap of the Q&A, including questions we weren’t able to get to during the live event.
What is driving the need for real-time processing? Why now?
A few factors have been at play – a massive explosion in data being generated today (the three years in this decade has generated more data than ever before), the perfect storm of technological advancements (AI, IoT, 5G, and social media) and, finally, the culture of instant gratification. All these have created the need to access and process information in real time.What are some of the key requirements enterprises have for real-time processing platforms?
While enterprises undoubtedly have the need to process data in real time, they also want to do it with the ability to easily scale on demand while providing data integrity and security. So, hybrid / multi / inter-cloud-based deployments, along with support for ACID principles, strong encryption (in-motion and at-rest), and role-based access controls are capabilities that enterprises are demanding of their data processing platforms.Can you give some examples of Fintech use cases where a unified real-time data processing
platform may have been used or could be used?
There are a number of use cases across the Fintech domain that have a need to consume and process streaming data in real time. All such use cases, and any others that require any form of analytics executed on such data, would benefit from a unified real-time data platform. Some such examples include:
*Asset Pricing – Pricing of complex or derivative products requires consuming streaming market data, updating individual asset prices in the complex product, and executing risk calculations to determine the appropriate asset price.
*FRTB & xVA – Cross-asset and different types of risk calculations require combining the latest positions data for multiple assets within the portfolio and executing what-if analysis for pre-trade calculations or conducting any form of post-trade risk profile and valuation adjustments.
*Post-trade Services – WIth T+0 becoming the standard that all financial institutions will have to comply with, performance, reliability, and accuracy become key in the post-trade processing, whether it is matching, validation, custodial services, risk assessment, or other activities all the way through settlement and clearance services.
*Regulatory Reporting – Validating trades and trade activities for corporate governance and regulatory compliance requires execution of complex rules against all trade transactions, as well as recording and reporting on the outcome of such analysis in close-to real time. Further, this validation practically always has to be done under strict SLAs to avoid unwanted audits and penalties.
*Risk Management – Computing position values post-trade, calculating cross-asset risk, and dynamically determining appropriate actions to mitigate any unexpected risks due to market movements is also a key use case for a unified real-time data platform. This requires processing incoming data from various market feeds, updating position values, and executing complex risk models in real time to minimize risk exposure and limit unnecessary margins and liquidity requirements.Can GridGain be deployed anywhere?
Yes, GridGain can be deployed anywhere – on bare metal on-premises, on virtual machines and in containerized environments, and in any public or private cloud or data center.
Can GridGain scale from on-premises to the public cloud?
Yes, GridGain can be deployed in hybrid, multi, or inter-cloud configurations. This means you could have a single GridGain cluster running in two private data centers, on-premises and in the cloud, or between two cloud vendor infrastructures, scaling on demand in and across any of these infrastructures. GridGain can also replicate across any of these infrastructures.
How does GridGain compare to or differentiate from the data processing solutions that the Hyperscalers (or cloud providers) provide?
GridGain is a cloud-native unified real-time data platform. The cloud vendors typically have a combination of solutions that together provide the storage and processing of data at-rest and in-motion. Solution developers take on the responsibility of integrating these solutions. GridGain, on the other hand, natively provides these capabilities in a single platform, thereby minimizing data movement between silos, limiting data integrity and security-related challenges, without compromising on enterprise-grade reliability and scale.
Does GridGain support ACID with strict consistency?
Not only does GridGain support ACID compliance and strict consistency, it also goes one step ahead and provides configurable strict or eventual consistency. GridGain also provides the flexibility to determine the consistency level per data cache. This way, enterprises can determine and configure their cluster appropriately for their specific use cases.
Can we execute AI models within GridGain?
Yes, not only can you execute AI models within GridGain, you can also use the high-performance, in-memory distributed computing capabilities of the platform to generate training data and train the models for AI workloads. GridGain, if also used as a transactional platform or a platform to store transactional data, can be configured to automatically extract features out of the streaming transactions and execute decision-making AI models on those incoming features, all in real time.
There was mention of use cases being implemented to MVPs in FinTalkr in four to six weeks. Can you please elaborate?
The FInTalkr platform is trained for financial advice but is easily extendable to other use cases. Implementing new use cases (eg Mortgage Hardship, Insurance Claims) is normally configuration and training data only. These use cases can be implemented in four to six weeks to MVP depending on the complexity. The company is also open to partnering with other organizations on these use cases.
How does data sovereignty work in FinTalkr?
Because FinTalkr runs GridGain on Openshift and is cloud-agnostic, the platform can be easily deployed to other regions in public, private, or hybrid clouds. GridGain’s persistent store capability supports isolation of data to the desired region to satisfy data privacy regulations.
What are the main features of GridGain employed within Fintalkr?
Fintalkr leverages many of GridGain’s native functionality including data and compute co-location through affinity co-location, partitioning, streaming analytics, clustering, in-memory data grid, persistent store, messaging, cache, and SQL queries. As mentioned in the presentation, the platform leverages grid services to build discrete microservices that can be easily deployed onto the GridGain cluster. These grid services run on either storage or compute nodes.
If there are other questions on the FIntalkr platform, can we reach out to the company directly?
Yes, you can reach out to James Wartho (CEO, Founder: jwartho@fifthlogic.com)
or Ashley Jeffs (CTO, Co-Founder: ajeffs@fifthlogic.com) directly with further questions.